Reinforcement Learning meets Federated Learning and Distributional Robustness
Prof. Yuejie Chi
13:00 (BST), July 5, 2023
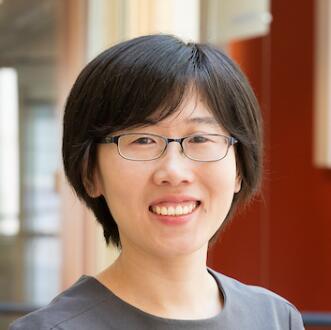
Abstract
Reinforcement learning (RL) is garnering significant interest in recent years due to its success in a wide variety of modern applications. However, theoretical understandings on the non-asymptotic sample efficiencies of RL algorithms remain elusive, and are in imminent need to cope with the ever-increasing problem dimensions. In this talk, we discuss our recent progress on understanding and improving the efficacy and resiliency of RL algorithms in tabular Markov decision processes (MDPs) through two vignettes. In the first part, we discuss how federated versions of RL algorithms allow collaborative learning using data collected by multiple agents in a distributed manner without central sharing. We propose an importance averaging scheme that only requires the agents to collectively cover the entire state-action space, thus unveiling the blessing of heterogeneity. In the second part, we discuss how model robustness can be incorporated to reduce the sim-to-real gap in practice via learning in distributionally robust MDPs (RMDPs). We uncover that RMDPs are not necessarily easier or harder to learn than standard MDPs: the statistical consequence incurred by the robustness requirement depends heavily on the size and shape of the uncertainty set.
Our Speaker
Dr. Yuejie Chi is the Sense of Wonder Group Endowed Professor of Electrical and Computer Engineering in AI Systems at Carnegie Mellon University, with courtesy appointments in the Machine Learning department and CyLab. She received her Ph.D. and M.A. from Princeton University, and B. Eng. (Hon.) from Tsinghua University, all in Electrical Engineering. Her research interests lie in the theoretical and algorithmic foundations of data science, signal processing, machine learning and inverse problems, with applications in sensing, imaging, decision making, and societal systems, broadly defined. Among others, Dr. Chi received the Presidential Early Career Award for Scientists and Engineers (PECASE) and the inaugural IEEE Signal Processing Society Early Career Technical Achievement Award for contributions to high-dimensional structured signal processing. She is an IEEE Fellow (Class of 2023) for contributions to statistical signal processing with low-dimensional structures.
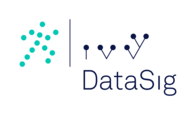
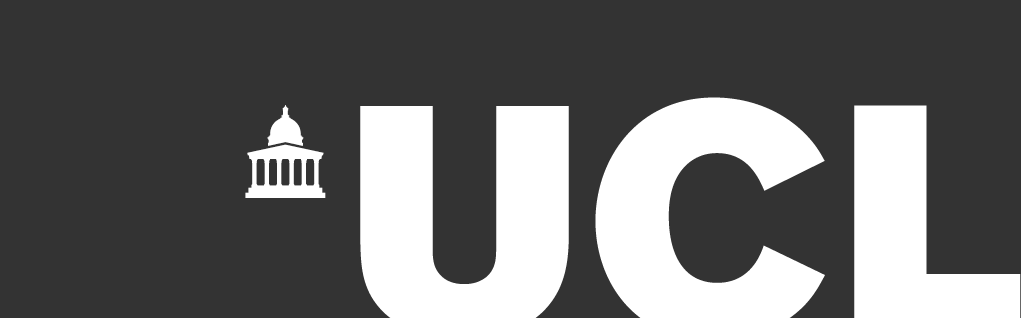
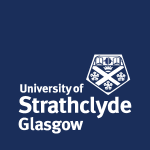