Dynamic pricing and learning with Bayesian persuasion
Prof. Shipra Agrawal
16:00 (GMT), Nov 30th, 2023
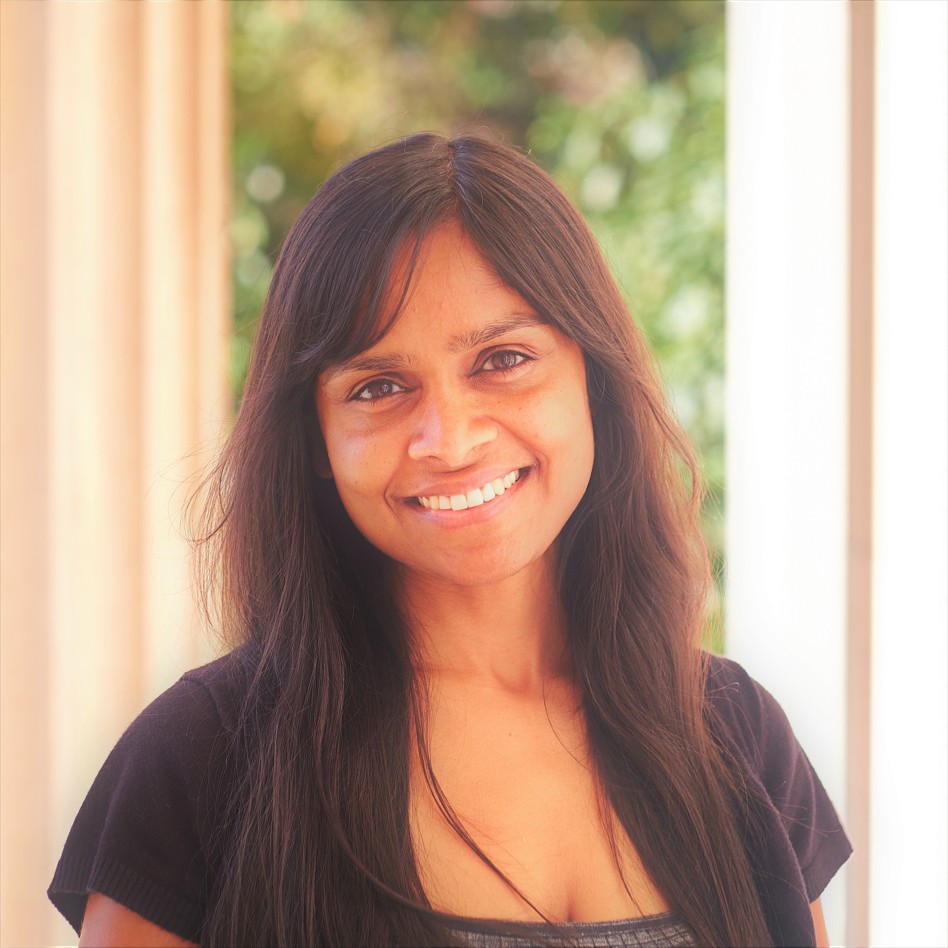
Abstract
Modern customers' decisions are intricately shaped not only by their own preferences but also by the associated product information and the offered price. We consider a novel dynamic pricing and learning setting where in addition to setting prices of products in sequential rounds, the seller also ex-ante commits to `advertising schemes’. Using the popular Bayesian persuasion framework to model the effect of these signals on the buyers’ valuation and purchase responses, we formulate the problem of finding an optimal design of the advertising scheme along with a pricing scheme that maximizes the seller’s expected revenue. Without knowing the buyers’ demand function, our goal is to design an online algorithm that can adaptively learn the optimal pricing and advertising strategy.
Our main result is a computationally efficient online algorithm that achieves O(T^{2/3}(m log T)^{1/3}) regret when the valuation function is linear in the product quality. Here m is the # of the discrete product quality signals and T is the time horizon. For constant m, our result matches the regret lower bound for dynamic pricing within logarithmic factors, which is a special case of our problem.
Our Speaker
Shipra Agrawal is an Associate Professor of the Department of Industrial Engineering and Operations Research at Columbia University. She is also affiliated with the Department of Computer Science and the Data Science Institute. She received her PhD in Computer Science from Stanford University in June 2011 under the guidance of Prof. Yinyu Ye, and was a researcher at Microsoft Research India from July 2011 to August 2015. Her research spans several areas of optimization and machine learning, including online optimization under uncertainty, multi-armed bandits, online learning, reinforcement learning, and learning in games and auctions. Shipra serves as an associate editor for Management Science, INFORMS Journal on Optimization, and Journal of Machine Learning Research (JMLR). Her research is supported by a Google Faculty research award (2017), Amazon research award (2017), and an NSF CAREER Award.
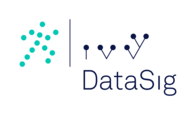
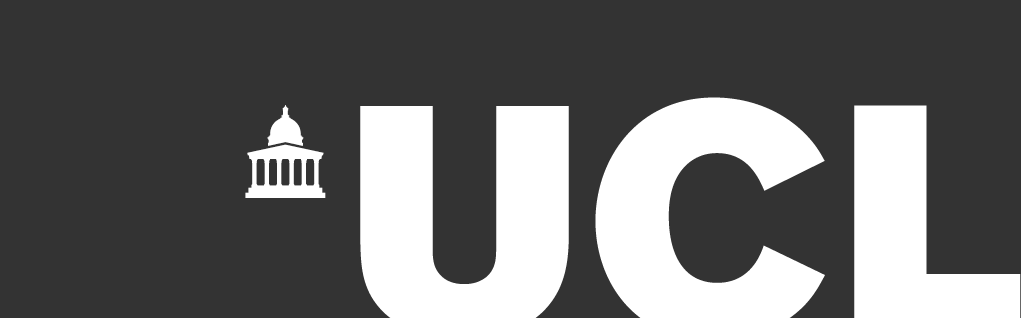
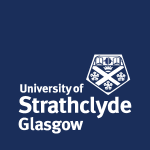