AI and the Future of Optimization
Prof. Madeleine Udell
16:00 (BST), Apr 24th, 2024
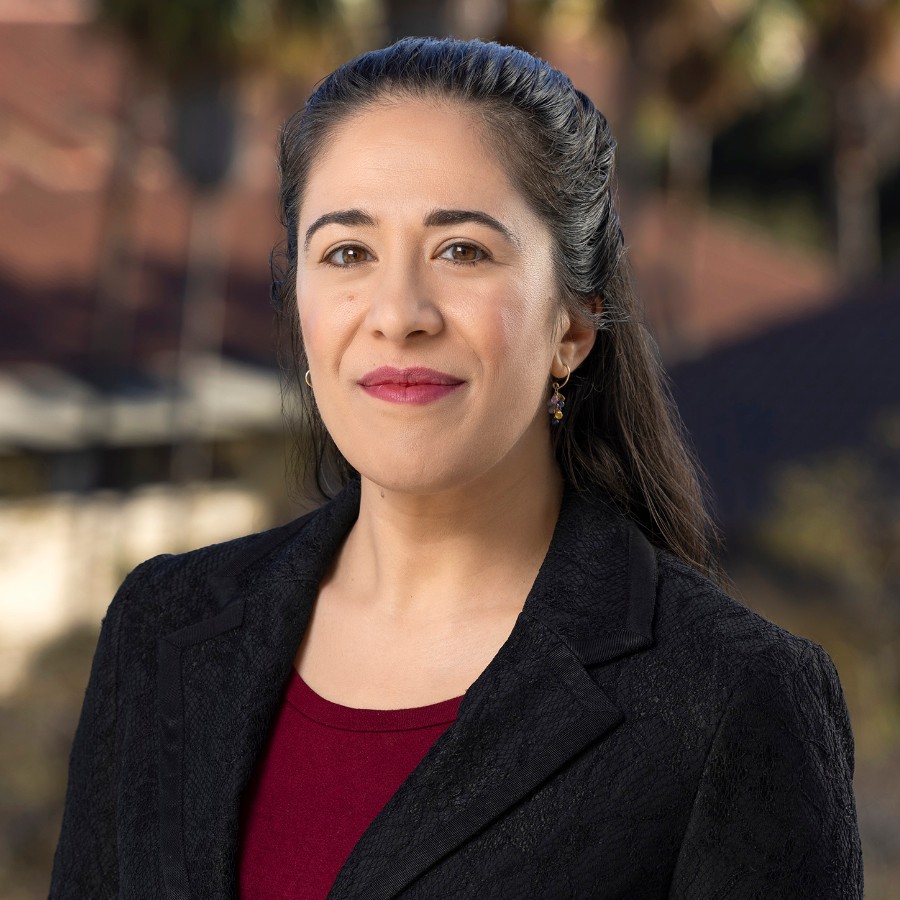
Abstract
Optimization problems are pervasive in sectors from manufacturing and distribution to healthcare. However, most such problems are still solved heuristically by hand rather than optimally by state-of-the-art solvers, as the expertise required to formulate and solve these problems limits the widespread adoption of optimization tools and techniques. As a glimpse of the future, this talk will introduce OptiMUS, a Large Language Model (LLM)-based agent designed to formulate and solve MILP problems from natural language descriptions. OptiMUS can develop mathematical models, write and debug solver code, develop tests, and check the validity of generated solutions. Experimentally, OptiMUS correctly solves more than 80% of benchmark problems, more than twice as many as a basic LLM prompting strategy. More broadly, we discuss the potential for LLMs in domains where accuracy and fidelity to real-world data is critical and strategies to augment and safeguard their performance.
Our Speaker
Madeleine Udell is Assistant Professor of Management Science and Engineering at Stanford University, with an affiliation with the Institute for Computational and Mathematical Engineering (ICME) and courtesy appointment in Electrical Engineering. She completed her PhD at Stanford in Computational and Mathematical Engineering and a postdoctoral fellowship at the Center for the Mathematics of Information at Caltech. Her research aims to accelerate and simplify large-scale data analysis and optimization, with impact on challenges in healthcare, finance, marketing, operations, and engineering systems design, among others. Her work in optimization seeks to detect and exploit novel structures, leading to faster and more memory-efficient algorithms, automatic proofs of optimality, better complexity guarantees, and user-friendly optimization solvers and modeling languages. Her work in achine learning centers on challenges of data preprocessing, interpretability, and causality, which are critical to practical application in domains with messy data. Her awards include the Kavli Fellowship (2023), Alfred P. Sloan Research Fellowship (2021), an NSF CAREER award (2020), and an ONR Young Investigator Award (2020).
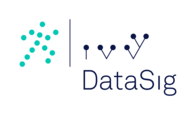
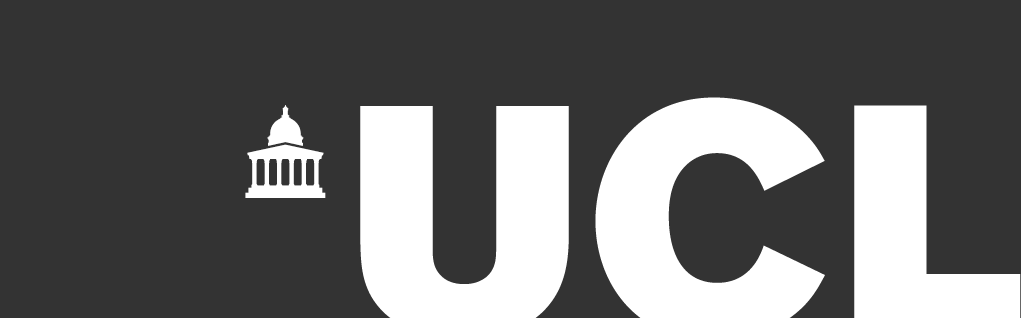
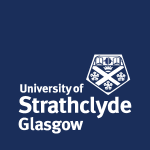