Contextual Selection of Conformal Uncertainty Sets for Conditional Robust Optimization
Speaker: Prof. Haojie Ren
Time: 14:00 (GMT), Feb 19, 2025
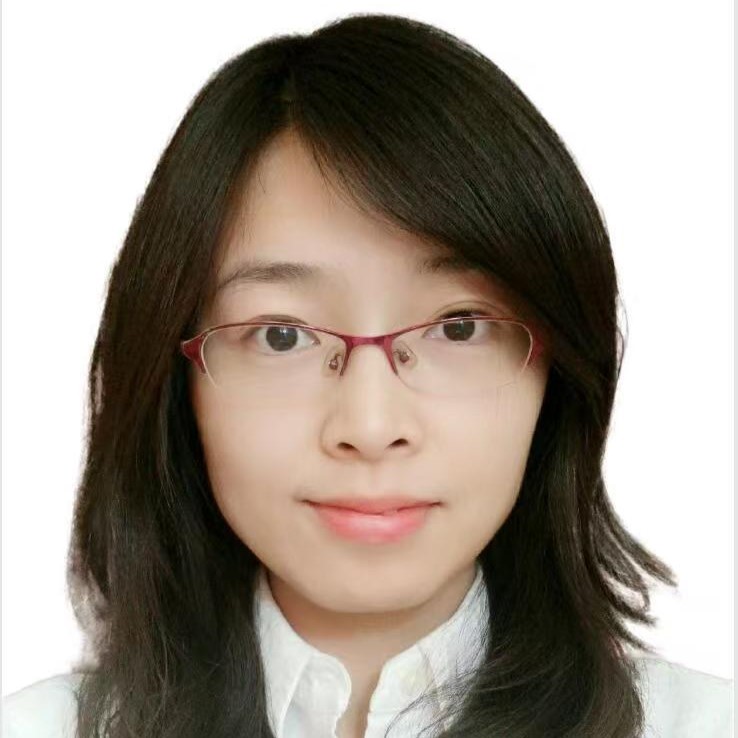
Abstract
In decision-making under uncertainty, conditional robust optimization ensures reliable performance by hedging against variability in uncertain parameters. While recent advances utilize conformal inference to construct uncertainty sets for machine learning models, downstream optimization outcomes critically depend on the choice of these sets a factor often overlooked in practice. This paper introduces a contextual selection framework that dynamically adapts conformal uncertainty sets to the specific covariates and requirements of a given optimization problem. By integrating conformal prediction with robust optimization, our method automatically selects uncertainty sets that balance robustness and conservatism, tailoring them to the problem's context while maintaining rigorous probabilistic guarantees. Theoretical analysis demonstrates that the framework achieves valid coverage and near-optimal solution quality. Numerical experiments across diverse applications show significant improvements in cost efficiency and constraint satisfaction compared to non-contextual approaches. By bridging statistical learning with decision-aware optimization, this work offers a principled data-driven paradigm for adaptive uncertainty quantification in real-world systems.
Our Speaker
Haojie Ren is currently an Associate Professor in School of Mathematical Sciences, Shanghai Jiao Tong University. Prior to this, she was an Eberly Postdoc Fellow in the Department of Statistics, The Pennsylvania State University, under the supervision of Professor Runze Li from 2019 to 2021. She received her B.S, M.S, and Ph.D. in Statistics from Nankai University in 2013, 2016, 2018, respectively. Haojie's research interests include conformal inference, machine learning, change-point detection and outlier identification.
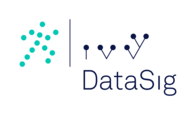
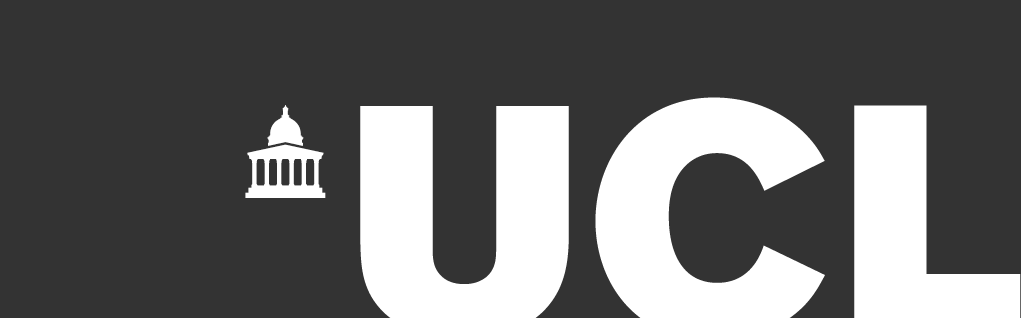
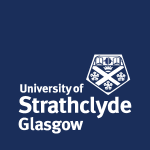