Machine learning in solution of inverse problems: a subjective perspective
Speaker: Prof. Marta M. Betcke
Time: 14:00 (BST), April 30, 2025
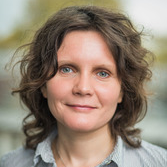
Abstract
Following the 2012 breakthrough in deep learning for classification and visions problems, the last decade has seen tremendous raise of interest in machine learning in a wider mathematical research community from foundational research through field specific analysis to applications. As data is at the core of any inverse problem, it was a natural direction for the field to investigate how machine learning could aid various aspects of inversion yielding numerous approaches from somewhat ad-hoc but very effective like learned unrolled methods to provably convergent learned regularisers with everything in between. In this talk I will review some on these developments through a lens of the research of our group.
Our Speaker
I am Professor of Scientific Computing in the Department of Computer Science at the University College London. I am also a member of Centre for Inverse Problems and Hawkes Institute (formerly Centre for Medical Image Computing). Between 2010 and 2013, I held an EPSRC Postdoctoral Research Fellowship "Image Reconstruction: the Sparse Way"at UCL to investigate the implications of sparsity on image acquisition and reconstruction in different modalities. Before coming to UCL in the end of 2009, I was a PDRA in the School of Mathematics at the University of Manchester working on novel X-ray CT scanners for airport baggage screening. My interest is in broad areas of Inverse Problems, Numerical Analysis, Scientific Computing and Machine Learning. In particular in numerical solution of inverse problems, tomographic image reconstruction, tailored PDE solvers for use in inverse iterations (PDE based forward problems), compressed sensing, sparsity and compression, applied harmonic analysis and machine learning. I enjoy working on mathematical problems which arise from real life applications. Presently, I am particularly interested in methods which rigorously combine numerical analysis with machine learning to leverage available data.
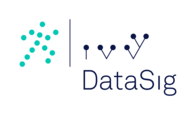
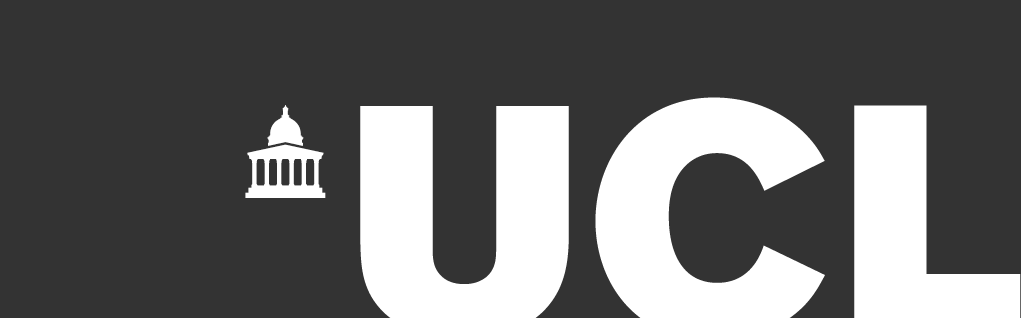
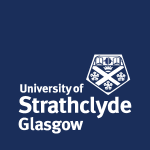